Trust Me, I'm an AI: Aaron Harris on the Future of Accounting
Attention: This is a machine-generated transcript. As such, there may be spelling, grammar, and accuracy errors throughout. Thank you for your understanding!
Blake Oliver: [00:00:09] Hello, everyone, and welcome back to the show. I'm Blake Oliver,
David Leary: [00:00:12] I'm David Leary-
Blake Oliver: [00:00:14] And we are joined today by Aaron Harris, CTO at Sage. Aaron, welcome back to the show.
Aaron Harris: [00:00:20] Yeah, thanks for having me. Always good to be here.
Blake Oliver: [00:00:22] Looks like you are at Sage Transform and coming to us right from the event. How's it going?
Aaron Harris: [00:00:29] I am indeed. Yeah. I just finished my keynote about an hour ago, so I am doing great because I got that out of the way and now it's nothing but fun from here on out.
David Leary: [00:00:40] That's good. So at the press conference earlier this week, you were talking about, uh, AI, but you also put it in context of, at this point, you've been around Sage Intacct for so long, right? It was your baby to begin with, and you think you'd be winding down your career and AI has, like, rejuvenated you. Like you're more excited than ever. Like you're not going to step out. Can you? Yeah.
Aaron Harris: [00:01:02] Yeah. I mean, I went through a period of time after I really understood the implications of large language models. I went through this period of time of wondering, you know, there is this is an entirely new era of technology. It is completely different than the way we've built things in the past. And I really did go through this, this sort of self introspection, like, am I still the man for the job? Uh, can I, can I lead the company through this? And, you know, a lot of sleepless nights, but when I really dug into it and started to learn and, you know, ironically, the thing I was sort of anxious about was teaching me. So, you know, with a lot of help from ChatGPT, it just becomes so exciting. And, you know, when you can really get into the works of the way large language models operate and just see the magic. It's it's just a great time to be a CTO. It's a great time to be in technology, period.
Blake Oliver: [00:02:02] I tell people it's a great time to be alive. It's a great time to be alive, period. Because like so much of what uh, I read about in science fiction as a kid is now coming to life. And like you, Aaron, I'm using AI every single day to help me learn how to do new things. David has a great example. David, what was your example you used?
David Leary: [00:02:24] I used it just to do one cell in Google. Google Sheet. I had to write a formula in one cell and it saved two people weeks a year from our team. Oh wow. Just just 111 cell. I had it created for me. Amazing.
Blake Oliver: [00:02:36] And something that we never would have had the capacity to do before. And it's interesting because we're using it. We're using it every day, but most people aren't. I survey accounting professionals when I do webinars, when I go to events, and my estimate is about 20% of them have tried it. Um, and it's understandable, right? Like it's not in the products we use. You have to go out and sign up and and use something new. Right. But you're you're changing that. Aaron, the big announcement at Sage transform is this new product, Sage Copilot, which is going to bring generative AI inside of Sage Intacct. Tell us about that.
Aaron Harris: [00:03:13] Yeah. So large language models have this amazing ability to drive, you know, very unstructured conversations. But the real power is that they can take what would be complex to code in the old traditional ways of coding and write the code itself and, you know, make some decisions about a sequence of, of steps they should take to complete some task. And so it just becomes a very different way of interacting with, with software. But I think more importantly, as the developers of software, it gives us quite a bit more power in how we automate the workflow within the software. So it'll it will, as you would probably expect, be available to answer questions like, what do you project my cash balance to be at the end of the month, but it will also do things like say, hey, since we last, uh, since you last logged in, I processed 18 invoices. And can you take a look at this one? Because I'm not sure that I coded it correctly or hey, take a look at this one because the amounts a bit off versus other invoices that we've seen. And so the whole idea is to get accountants out of that routine work of doing and essentially let copilot do the work where where the accountant is doing the reviewing. Mm.
Blake Oliver: [00:04:36] There's a great example in the video on the Sage copilot web page where the accountant, the user, uh, gets a prompt from the AI that says, hey, Melissa hasn't submitted her time sheet yet. Would you like me to remind her? And then the user says, yes. And then the AI copilot drafts the reminder and says, you want me to send this? And then the user says, yes. And it's sent.
Aaron Harris: [00:05:02] Yep. Yeah. So we we talk a lot. I mean, my whole. Keynote today was on how do we use technology to eliminate the monthly close? And one of the biggest problems in getting the monthly close done is having to chase people to submit their timesheet right, or to approve or review some transaction. And so it sort of sounds like a little thing, but if you can hand that responsibility off to copilot who doesn't just sort of manage the communication, but also knows, you know, what's happening with the close, what are we still waiting on and who do I need to chase? Um, so that's that's sort of an overlooked value of this is actually not what it does for the accountant. It's it's an ability to reach out to people outside the accounting team. To where they're, you know, where they're a dependency on a workflow and just make it easier for them to do what accounting needs them to do.
Blake Oliver: [00:05:55] I love this example because it's something that's such a pain chasing people for what you need. Can you give me any other examples of what users who are testing Sage Copilot are using it for? What are they saving time with?
Aaron Harris: [00:06:08] So, uh, I mean, a lot of it is around that communication. So drafting an email to send to a customer to, um, you know, to to ask them to pay or to provide some, some updated compliance information. One of the use cases that's, um, really picking up is actually accountants collaborating with their clients to get the needed documents and information that they need for an engagement. So, for example, if it's a tax return, you know, having having copilot run that process of gathering the information that's needed from the clients, it's going to vary across different types of users and accountants and practice versus a CFO versus a small business owner or a controller. Um. There's, you know, there's there's obviously tons of enthusiasm around the ability to ask copilot for an answer without having to run a report. One of the things that I didn't expect, but has turned into a huge, huge fan fan favorite is and this is not intact. This is our Sage 50 product and Sage 50. We've got something like 900 canned reports. There's a report for everything. Um, and of course, one of the one of the top support questions that the team that's supporting Sage 50 gets is what report should I run? And so, you know, this isn't a copilot thing. We've just used a large language model. We've trained it on all the report definitions. We built a simple interface that essentially allows the user to ask a question. And in response, we run one of those those 900 reports, uh, people love it.
Blake Oliver: [00:07:53] And you could conceivably use it to generate custom reports based on a text prompt. I could say in natural language, I want to see every paycheck by every employee for this date range, with these fields broken out in columns. Yes, Jen, I could totally do that. Right?
Aaron Harris: [00:08:10] Absolutely. It can do that.
David Leary: [00:08:12] So can you. Oh.
Blake Oliver: [00:08:13] Go ahead. Go for it, David.
David Leary: [00:08:15] Go for it. Can you explain a little bit on the background because I, you mentioned that you trained it. You've kept data secure and siloed out and then explain because it's copilot is this you're built with Microsoft Copilot. I know you guys also have to do stuff with Amazon. Like just clarify what you built and what the actual offering is.
Aaron Harris: [00:08:32] Yeah. So what we're getting from Microsoft is access to GPT four through Azure. And behind that, a lot of support from Microsoft in in how we actually interact and get, you know, our needs more on the sort of the scale and the performance side of things. So we use GPT four as that, what I call the orchestration or the conversation layer. But but the way you build something like copilot. Is you rely on the large language models at that tier. But then fundamentally what you have to do is build skills for the copilot to do the things that you envision copilot doing for your customers. And so we've got a set of models that we've developed for doing cash flow forecasting, for example. And we've got a set of models we've developed for we have 27 models just for processing an invoice. And that's where we spend, you know, our time, you know our research. It's on these very specific accounting related problems. And the way this works is a customer will ask a question. And copilot will then break that down into what steps it needs to take to complete whatever question it is, which may include making an API call that we've we've we've created a skill for it to do that, access some documentation. We've created a skill for that, use a model we've developed to do a forecast. And it it's it's so with with partners. Yesterday I did this crazy thing. I showed them what it's like to debug copilot because it's such a radically different experience for, for an AI engineer to not step through code. But to actually have a conversation with copilot in in the process of debugging copilot. It's absolutely crazy.
Blake Oliver: [00:10:29] We're working on using AI to help us generate continuing education courses and perform compliance at earmark. And so I've been experimenting with the ChatGPT platform, the API, the the playground. And, um, it's it's been really interesting experiencing it for myself because, for example, we we have these long transcripts of podcast episodes and we want to do stuff with that. But these transcripts are larger than the context window for ChatGPT for turbo. Right. Because they're so long. So the question then becomes, and I guess this is like a programing or coding question is how do I break down this transcript into steps or to pieces that I can then process to get the result I want, which I can't get because the context window, the amount of text I have is too much, right?
Aaron Harris: [00:11:19] Well, there's a couple I mean, there's a couple of approaches to that. Um, you know, one is essentially chaining, you know, chaining the conversation, you know, through these things. Yeah. Uh, but this is where retrieval, retrieval, augmented. Um, what's the G stand for? Rag retrieval. Augmented generation comes into play where essentially, instead of feeding the data into the large language model where it puts it into its vector data store, instead, you're giving the large language model a skill to interrogate and interact with data. And so your prompts can be pretty simple. In this case, you're just asking AI something about the transcript. And then what it's doing is it's actually going out and and analyzing the, you know, the data that, that, that you've stored for it.
Blake Oliver: [00:12:10] Right. But you have to do it in this, like, you have to really think about how you do it, because it costs money to send a lot of data into these APIs. Right. So your prompts need to be as streamlined as possible. And that's what you've done with these assistants. You've built. Right. As you've you've trained them, you've tuned them up. You've made them efficient and fast. Yeah. It's yeah it's fascinating.
Aaron Harris: [00:12:34] It it's it's really fascinating to see the code behind the scenes because a lot of that is what we call system prompts. So, you know, this is human language. It in the code instructing copilot on a way to behave or some skill that it has. And, you know, there is a process with models like GPT four to actually train these as a layer on top of the model. So, so there are approaches to actually building those system level prompts into the model, so that you don't have to incur the costs of, of the tokens every single time you ask a question.
David Leary: [00:13:13] So speaking of cost, uh, it was mentioned in the press conference that there's going to be a charge for this product, um, because you're going to produce so much value. Is this correct? Did I hear it correctly? And give an example, the value copilot could create.
Blake Oliver: [00:13:27] And I was thinking as I play around with it myself, there has to be a charge because I'm seeing my bill rack up and like some of these, like a typical prompt can cost, you know, $0.40. So, uh, and I could imagine people using this a lot if it becomes really effective. So yeah.
Aaron Harris: [00:13:43] I is not cheap. Um, what's what's guiding us here is actually making sure that in the cases that we support with copilot, that there is real value. And a big part of that is identifying where the pain is today, where, you know, copilot can come in and provide an answer. Um. I don't know exactly how we're going to price this. I love what's going on right now with my. This is.
Blake Oliver: [00:14:10] So strange. We have to bring all this out because this happened twice now. You gave a thumbs up and oh, did. And, well, your thumb it. There must be some AI going on with the video here, because your thumb went up and it gave a thumbs up on your screen, like on our video for you. And then we just saw, like an explosion, fireworks behind you. So there's some AI in between us right now that's adding these emoji reactions.
Aaron Harris: [00:14:36] That I'm not clicking some buttons to say, give him a thumbs up to the fireworks. It's really hard for somebody who speaks with their hands to know that I make the wrong gesture. I can add some flowery, you know, exciting, uh, animation.
Blake Oliver: [00:14:48] Can you try the thumbs up again? I want to see if this is on your end or, like, what is happening.
Aaron Harris: [00:14:52] I've never been able to reproduce it.
Blake Oliver: [00:14:54] It's so strange. But, I mean, it's it's a great example of what we're talking about, which is like the video, like maybe still images every now and then of this video are being fed into an AI, which is then interpreting your hand gestures and then deciding whether or not to insert some sort of graphic into the feed. Yeah, we're living with it.
Aaron Harris: [00:15:13] Yeah for sure.
Blake Oliver: [00:15:15] So yeah, I completely forgot where we were going, but maybe you can get us back on.
Aaron Harris: [00:15:18] Track about pricing.
Blake Oliver: [00:15:20] Pricing. Okay.
Aaron Harris: [00:15:20] Um, yeah. Sort of the conventional wisdom right now is that the commercial opportunity with generative AI is in, um, specific applications. It's not in the more general, uh, capabilities. You know, it's AI that can do, you know, specific tasks in an industry is really where the value is going to come from. We know that we're going to bring a lot of value. We're focused on, you know, these these cases that, you know, where there is pain that we've identified how we're going to price this, I don't know. Uh, you know, there's lots of ways we might do this, but fundamentally, you know, we're we deeply believe in the value that we're creating. There is cost to it. So there will be some price yet to be determined.
Blake Oliver: [00:16:06] I like the way you're approaching it, though, which is let's create value first and then figure out how we charge for that value as opposed to there is an order here. Yeah. And I feel like just to pick on them because everyone's picking on them. This week Google did it the wrong way, which is let's create Gemini and then start charging people even to access it before they've even had a taste of it. Right. And then of course, everything goes haywire, which is, you know, not even really related, but maybe just sort of like points at the dysfunction in there.
Aaron Harris: [00:16:34] Um, well, I mean, there's a there's a principle in the startup world that you don't have a product that people want to use until you've figured out pricing where they want to pay.
Blake Oliver: [00:16:44] The other thing I like about your approach is you have, uh, tuned up specific models for specific use cases where, you know, the AI is going to do a good job. So you're like, you're going to give get a good experience because this is not just let's expose all the data in Sage Intacct to a general language model and then see if people get good results. You're actually trying to yeah. You're trying to ensure a good experience. Yeah.
Aaron Harris: [00:17:11] Large language models are not good at math. I was I was explaining this to somebody the other day. Large language model works off its memory. Right. If it's if it's seen a math problem with the answer, uh, it'll be able to give the answer. If it's seen that math problem with a couple of variations on the answer, you might get the answer that isn't the right answer. And so the first principle of preventing AI hallucinations is to not use a large language model for something where there is a deterministic answer, right, where where there's better technology. And so, you know, the way that we build this, there's very there's a there's a focus on knowing when the large language model should hand off to the skills that we give it, that are in many cases built around traditional programing techniques like SQL and API invocations, where you're going to get right a a consistent answer every single time, right?
Blake Oliver: [00:18:06] Yeah. This is one of the reasons why I caution accountants not to use. Chatgpt. Off the shelf for reconciliations or for converting bank statements into CSV files, to import into your accounting system, or asking it to do some sort of like mathematical stuff because it's a statistical model, which means that you're going to get a slightly different answer. And when our profession says it needs to be to the penny, you can't risk it not being accurate, right? It has to be the same answer every time. Yep. But so how do you solve that. So you you train the model to like use a calculator program or script instead.
Aaron Harris: [00:18:47] We yeah we use a technology called Lang chain. Um, it's, it's a pretty popular framework for using large language models to I call it orchestrate, but, but essentially orchestrate, you know, a series of work to complete some task. And what Lang Chain does is it, it breaks some requests down into the steps. And then what what we've done is we've done a lot of work to make sure then that the large language model knows. Which skill to use to do what, and some of these skills are narrow task based AI models, whereas some of them are, you know, very traditional services like like, you know, SQL to a database. So that's, you know, that's really more in the programing that we do around the large language model. You know, there is tuning, right? There's tuning in various aspects of this. Um, but um, yeah, it's, it's the framework is called Lang Chain. People should check it out. There's a, there's a product called auto GPT which uses that technology. It's it's it's pretty fascinating what people are doing.
Blake Oliver: [00:19:59] What about these concerns that AI is going to replace human accountants. Now that you've integrated this tool into the product? It's doing these little tasks for now. But we previously discussed, um, last year, this idea that the AI is going to become like. A staff accountant in your team, right? Like so. If I'm a staff accountant working at an organization that uses Sage Intacct, do I need to be worried that Sage copilot is going to become my coworker and then take my job?
Aaron Harris: [00:20:28] Well, I guess I would start with nobody wants to do the stuff that AI is going to be good at, you know, doing all the data entry and spotting errors. Um. But I go back to 1980. So 1980 was the first spreadsheet Visicalc. There were 339,000 accountants in the US thereabouts. And if you think about the technology available to accountants at the time, it was a ten key with a roll of white paper. And, you know, they would spend hours totaling up columns and then, you know, cross-referencing and making sure the T accounts, you know, work out. And the trial balance is accurate. With Visicalc. Suddenly you could do that in a spreadsheet and you'd go from hours to seconds. And I think we're going to see something similar, you know, when we really embrace this and we're going to look back on things like doing data entry, where it's taking seconds now and, you know, wonder how we were ever doing this, this, this manually. And the industry did what it does best. Right? It sort of moved up the value chain in how it serves its clients and the businesses that they, that they work for. Um, and that's there's one there's one other argument that that I think is super important here. And that is the more we use technology to automate, the more we need human trust to, you know, to to sort of stand by the results of that automation. I sort of joke that, that we're never going to accept an audit report signed by an AI. Uh, and so the accounting industry and its ability to provide third party assurance will only become more valuable in the future as we're depending more and more on technology.
Blake Oliver: [00:22:10] Yeah, more automation, more tech involved in how the data gets into the system. You still got to have somebody who understands how that all works and can sign off on it.
David Leary: [00:22:21] Because it's not gonna directly replace an individual. It's going to replace 20% of an individual's annoying crappy work, and somebody else is 20% of annoying crappy work and somebody else's 20%. And your org. That might add up to a full team member, but. Right.
Aaron Harris: [00:22:35] Yeah. So in accounts payable automation, um, you know, what's happening is they're spending more time managing their vendor relationships. Yeah.
Blake Oliver: [00:22:43] Actually following up.
Aaron Harris: [00:22:45] Yeah. And actually something I was kind of surprising to me. Uh, people want to pay their vendors faster. Yeah. Uh, because they really rely on having those good, healthy relationships in their supply chain. Yeah. And so just achieving that outcome has been super valuable to people. And, you know, the biggest stories that we're hearing from customers are really that as they're growing, they're not having to add staff to processing invoices. Right. They're adding staff to do more strategic things.
Blake Oliver: [00:23:17] Aaron, thank you so much for your time today. We've been talking with Aaron Harris, CTO at Sage, all about Sage Copilot and the AI that has come in to Sage Intacct and other Sage products soon. Is this available to everyone now or will it be available soon? What's the situation?
Aaron Harris: [00:23:33] Yeah, so we're making it first available to our Sage Accounting and Sage Accountants products in the UK market. We will spend some time making sure that we've got a good product there, and at some point, probably in 2025, we'll bring it to intact and other products. But I can't give you any specific dates yet.
Blake Oliver: [00:23:53] All right. Sounds good. We'll stay tuned. Aaron, uh, great talking with you and hope to see you again soon.
Aaron Harris: [00:23:58] Thanks for having me.
Creators and Guests
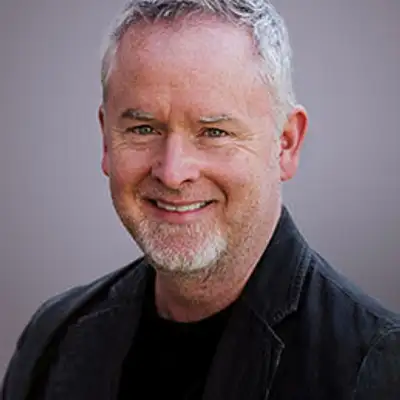
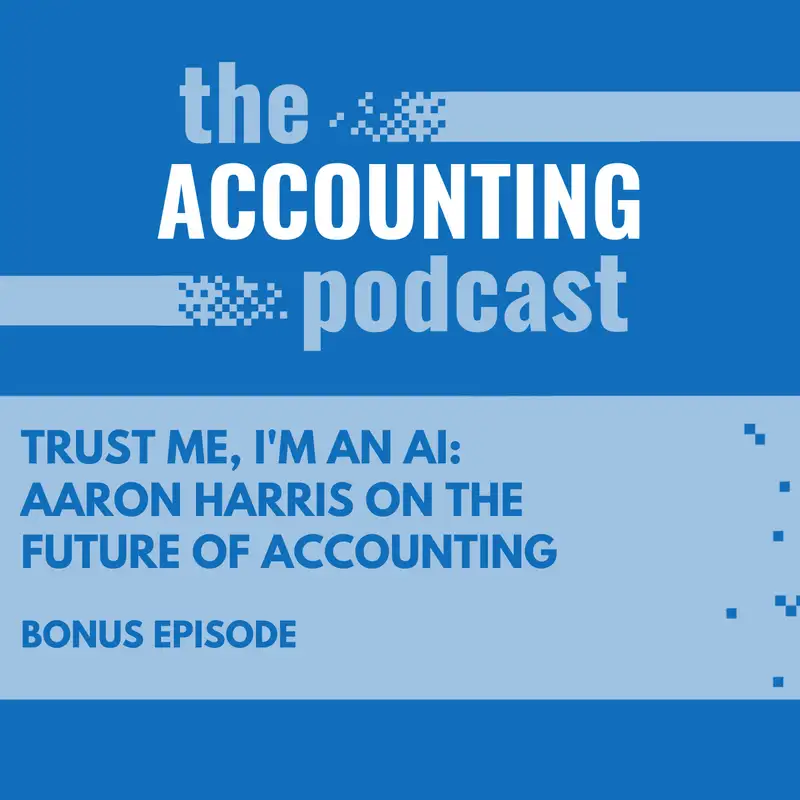