Automating SOX Audits with RAG
Attention: This is a machine-generated transcript. As such, there may be spelling, grammar, and accuracy errors throughout. Thank you for your understanding!
Blake Oliver: [00:00:00] Hey, everyone. Blake here. Today we're exploring the cutting edge of AI in auditing with a guest who represents the future of our profession. Josh Persky is a recent college graduate working in audit at a large accounting firm. He's also a listener of our podcast who reached out to share an AI project he's been working on in his spare time using a technique called retrieval, augmented generation, or Rag for short, Josh has created a program that can instantly query hundreds of pages of PCAOB audit guidelines. But that's just the beginning. Josh believes that with AI, we could automate up to 99% of staff work for internal controls testing in Sox audits. Stay tuned for my conversation with Josh. And hey, if you're working on an exciting AI project like Josh, I want to hear about it! Send an email to The Accounting Podcast at earmarked me that's The Accounting Podcast at earmarked me. Now let's dive in Hello? Josh Poresky. Hi. Nice to meet you.
Josh Poresky: [00:01:04] Nice to meet you as well. So I just graduated from college last year. As of today, I completed my first year at RSM. I work in the Sox audit team. It's called technology risk consulting.
Blake Oliver: [00:01:16] You mentioned this Rag acronym. What does that stand for?
Josh Poresky: [00:01:20] Let's say use ChatGPT as an example of a popular AI program. Right now it's trained on a very large majority of the internet. Openai has taken information from a bunch of different websites and used it to train this bot, and by training, it essentially does a what word has the highest chance to follow another. So if it reads a bunch of documents on sports from 2000 to 2020, it'll see that the Patriots were the greatest sports team in the history of the world. And that's how it functions. But what the Rag program does is that instead of using ChatGPT trained on the entire internet, you just give it. It's knowledge base. So it stands for retrieval augmented generation.
Blake Oliver: [00:02:04] So so I say to ChatGPT, I don't want you to focus on your whole knowledge base. I want you to focus on these specific documents that I'm providing you.
Josh Poresky: [00:02:12] Exactly.
Blake Oliver: [00:02:13] So you're doing Sox audits. What's an example of this?
Josh Poresky: [00:02:17] One thing that I've built that I think is pretty cool is I've taken the PCAOB audit guidelines and put that into a Rag program. Let's say we feed this bot a scenario, okay, there's a password control, and they don't have these two things enabled. Would that be a problem with the PCAOB. And it'll tell you based on the 665 pages of information, if yes, that would be an issue or no, it wouldn't be. And then you can ask follow ups, okay. But they have this compensating control. Let's say for example, they don't require an uppercase letter, but they require the password to be at least 15 characters long. Would that satisfy the pcaob's requirements. And it'll say yes, and give a short explanation as to why or no as a short and a short explanation as to why I love that.
Blake Oliver: [00:02:58] Because what's the alternative? In the past, you would have had to do keyword searches in this document. How would you answer that question?
Josh Poresky: [00:03:07] Typically by going to a senior manager.
Blake Oliver: [00:03:11] Somebody who just knows it.
Josh Poresky: [00:03:13] Someone who just knows it, which there's a lot to learn. It can take a long time to know this thing inside and out. So in that case, I think it saves a lot of time. It saves communication time. It saves the length in communications. I ask my senior question, she answers, or the answer is two hours later. There's that time. There's also just the time it takes to ask the question and the time it takes for them to respond.
Blake Oliver: [00:03:33] So can we see this?
Josh Poresky: [00:03:35] I called it the document querying app.
Blake Oliver: [00:03:37] We can work on that name.
Josh Poresky: [00:03:40] Exactly. We perform testing to make sure that management is adequately reviewing their own users access, to make sure no one has inappropriate access to make changes they shouldn't be making. So a sample question would be I have a user access review that is not signed off on five minutes with this. Consider an issue by the PCAOB. Pcaob is the government governing body of the Sox audits.
Blake Oliver: [00:04:12] I have a user access review that is not signed off on by management. Would this be considered an issue? And the answer is yes. A user access review that is not signed off on by management would be considered an issue by the PCAOB.
Josh Poresky: [00:04:25] And I can ask a follow up question such as what if there is a compensating control? A compensating control would be something along the lines of, oh, they didn't sign off on the user access review, but within the review they have a list of comments suggesting that they still performed the review.
Blake Oliver: [00:04:45] So in this case, we got the answer. If there is a compensating control in place for the user access review that is not signed off on by management, it may help mitigate the issue, but it is still important for management to provide the necessary sign off as required by the PCAOB.
Josh Poresky: [00:04:59] So in that case, instead of necessarily being an issue, it might be something along the lines of a process improvement. Again, it would take a couple more iterations to get exactly into it. And I'm not totally sure on a great example of a compensating control for something like that. But yeah, it's pretty quick. It's pretty good. So if I go and ask a question like who is Tom Brady? Typically ChatGPT would have a pretty good answer for that. He's been around for a while.
Blake Oliver: [00:05:28] Chances are, ChatGPT has come across some blog posts and some news articles about Tom Brady.
Josh Poresky: [00:05:34] It says Tom Brady is not mentioned in the provided context information.
Blake Oliver: [00:05:37] Got it. And so that prevents hallucinations too right? How do you deal with that? How do you deal with like making sure that the answer is is factual.
Josh Poresky: [00:05:46] I just finished making this yesterday. I haven't had a ton of time to double check it, but what I've tested it on is stuff I already know the answers to and everything I've asked. It's been right. So let me ask you this.
Blake Oliver: [00:06:01] Chatgpt has this concept of custom Gpts, where you can create an AI agent inside of ChatGPT. If you're on the team's plan and then you can upload documentation to it. So I could actually do that. I could take whatever 600 page document I need to analyze, and I could upload it, and then I could start querying it that way. How is what you're doing different than the custom GPT or is it similar?
Josh Poresky: [00:06:27] So it's definitely similar. I haven't tried uploading 600 pages of a PDF to the custom GPT to train it. I don't know if it actually could. So as a part of the process for it to learn the information I supplied to it as a part of the Rag program, it takes all 600 pages, and then it breaks it down into bite sized chunks. So roughly think about like 5000 words or so, and then it'll break all 660 pages into 5000 word chunks, and then it'll index it and squish it all together in a way that it's able to understand it That makes the data size much more manageable and it can better understand what's going on rather than the agents when it tries to read everything at once, it's a little too high level, and it won't really. The context will still be there, but it'll miss a lot of the specific details.
Blake Oliver: [00:07:18] So this way you get higher quality answers because it's not searching the whole document every time you make a query.
Josh Poresky: [00:07:23] Exactly. And that's why it can be almost instantaneous. And the agents, one I don't think would even work on this much data, I don't think. I haven't tried it.
Blake Oliver: [00:07:33] I've run into issues with that in the past. So I totally imagine that like a 600 page document might be too much. So how did you build this.
Josh Poresky: [00:07:40] Youtube Stack Overflow? So I built out a program in an application called VSCode. It's just like a code editor. It's pretty solid. I use the GPT API to the process I described earlier of the chunking with the words, and then the indexing. I use the API to do that, and then it also uses the API to then process that information to return output.
Blake Oliver: [00:08:05] So I noticed it doesn't cite a page number or anything like that. Is that something that you could do? Because I would want to know where in the document can I go to find this information.
Josh Poresky: [00:08:15] Oh, that's a good question. It depends on if the page numbers are in the text file or not. They might be, but I might be able to add them in even if they're not. That's a good question.
Blake Oliver: [00:08:28] I'm thinking like if I'm a manager and you're giving me this answer, I would want to know, where did you get it from? I wouldn't just trust the LLM.
Josh Poresky: [00:08:35] That's a good idea. Yeah, I'll try to add that in. Thank you.
Blake Oliver: [00:08:38] Well, I'd love to know if it works. I imagine it could, because when I take a PDF and I just upload it to the ChatGPT chatbots, I can then ask it, where did you get this information? It'll come back with a page number.
Josh Poresky: [00:08:50] The larger vision for this is to be able to take a like a piece of audit evidence. Let's say password configurations. Is the password at least eight characters long? Does it require uppercase lowercase letters that Letters require special characters and then test it. Say hey program, does this image fulfill these requirements? The requirements I just listed. And then it would say yes yes, no. Yes. And then send that analysis over to the Rag program and say, would this evidence be enough to pass the audit. And then you have the full loop of does it pass our requirements and does it pass the Pcaob's requirements? And I have these right now in two separate programs. I haven't linked them together yet, but that's the next step.
Blake Oliver: [00:09:38] So you can automate the testing of the evidence. You just have to get somebody to put the screenshot in to the program.
Josh Poresky: [00:09:48] So you need to have the file ready to upload. All you need to know is the file path on your computer.
Blake Oliver: [00:09:56] So user uploads the file. And then your program will basically test that for if it meets the standard or not. And that is something that a human has been doing in a check checkbox kind of situation. How much time do you think that could save per.
Josh Poresky: [00:10:12] Engagement over a time frame?
Blake Oliver: [00:10:13] Well, I guess the question is like how much time on a single test? How much time does it save? And then I guess, could we estimate how much time it's going to save us across, like an entire Sox audit?
Josh Poresky: [00:10:25] So across an entire Sox audit, I'd say it should be able to save us about 99% of the time for a given associate. I'd say it should be able to save a close to 1000 hours a year.
Blake Oliver: [00:10:39] That's crazy.
Josh Poresky: [00:10:40] It's unbelievable. It's really good at some things and not as good at other things right now. It doesn't have an awesome ability to take information from one document and compare it to information from a different document. It needs to be within the same one. But that's just a matter of OpenAI updating their models. And I think two years from now, I think it'll actually be at the 99%.
Blake Oliver: [00:11:05] So where do you see this going?
Josh Poresky: [00:11:07] So where I see it going is we have the audit procedures. The audit test steps that we need to ask the evidence. So for example for the passwords does this password configuration require eight characters. We take that as a test step. We take the audit evidence as the document that we're analyzing in the back end. I have a Rag program trained on every single password configuration that has passed, and another one on trained on every single password configuration that has failed. We're then able to take the audit evidence, ask it that question, and then based off of all the thousands of previous password audits, it will then be able to determine if this thing passes or fails. And I think that'll be the full loop.
Blake Oliver: [00:12:00] Automating the Sox audit.
Josh Poresky: [00:12:02] I'm sure that process will then have to be audited itself. Someone's got to sign off on it. There's not much visibility into how these programs work, though. I don't know how something like this can be audited, because the part of the part of the program that performs the analysis is five lines of code, four lines of code. It's not something that anyone has any visibility into. It's just it's a black hole you can't see. I guess the code in English is saying like, hello ChatGPT, this is the information being passed in. This is the question we want to know. And then the output line is just like print answer like there's no visibility. So to audit these things is a big reason why. It might be a while before we can actually see these implemented in an official accounting way.
Blake Oliver: [00:12:51] Well, you know what I would suggest adding into your program is for every answer, ask ChatGPT to provide its reason. Its explanation for that answer. It's still a black box. You don't know how it got to that answer, but the explanation might be enough to accept the answer.
Josh Poresky: [00:13:09] Oh, that's a good point. I initially wrote it to return as little information as possible, because I didn't want any kind of like opinion in it, but I think that actually might be helpful.
Blake Oliver: [00:13:22] So the reason I know this works is because we create CPE courses using AI, which create multiple choice questions, and the question has to have a question Stem. It has to have four answers. One is correct and three are wrong. And it has to have feedback for each question answer explaining why it is correct or why it is wrong, and that feedback is what allows us, when we review the question, to evaluate whether it's correct or incorrect. In combination with listening to the episode, it really is helpful. Give it a try and you might be impressed. Yeah.
Josh Poresky: [00:13:57] Oh yeah, definitely add. That also just helped me like test the thing out to see exactly how it's like what it's actually like.
Blake Oliver: [00:14:02] How is it thinking. Right.
Josh Poresky: [00:14:03] Yeah. Yeah, yeah.
Blake Oliver: [00:14:05] This is really neat. Josh, thank you for taking the time to show us what you're working on. I'd love to follow up with you when you got version two or whatever. You know, whatever changes you've made. This is such a great example of AI in audit, and I agree with you. I think it will automate 99% of the testing someday.
Josh Poresky: [00:14:23] Oh, it definitely will. It's just a matter of when. I mean, the technology is there. Like we can like it's physically possible. We're just not allowed to use it.
Blake Oliver: [00:14:32] But we will when the partners see just how much time we can save, we'll figure out how to get around the compliance issue. It's coming. And especially like you can, if you had the resources, you could actually run a LLM like on a server. Oh, totally. Hey, Josh, thank you for chatting with me.
Josh Poresky: [00:14:50] Thank you so much. It was great talking to you. Hey, hey.
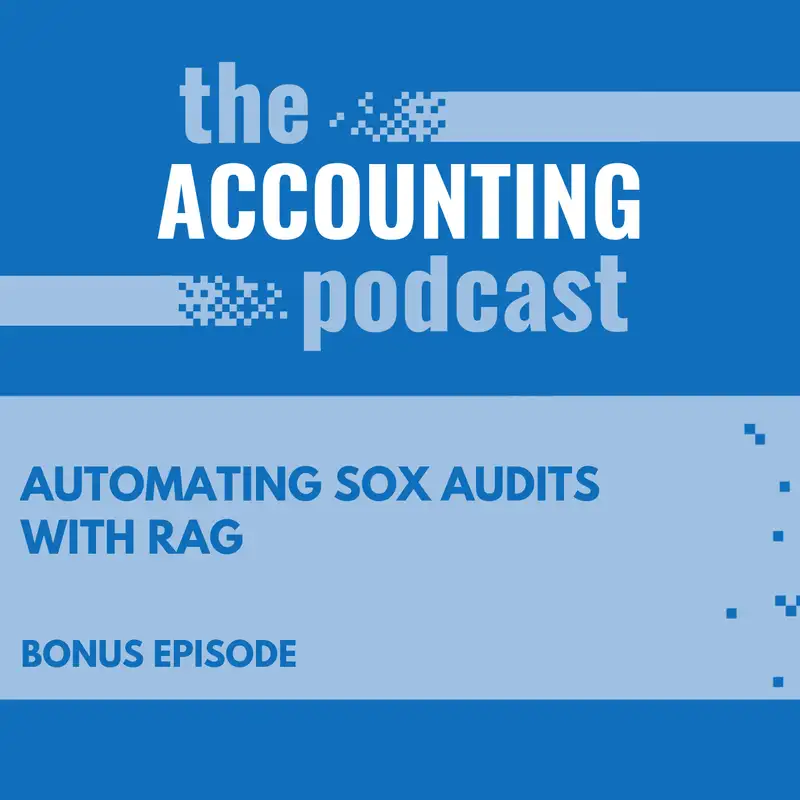